Applications of AI and Machine Learning in Venture Capital
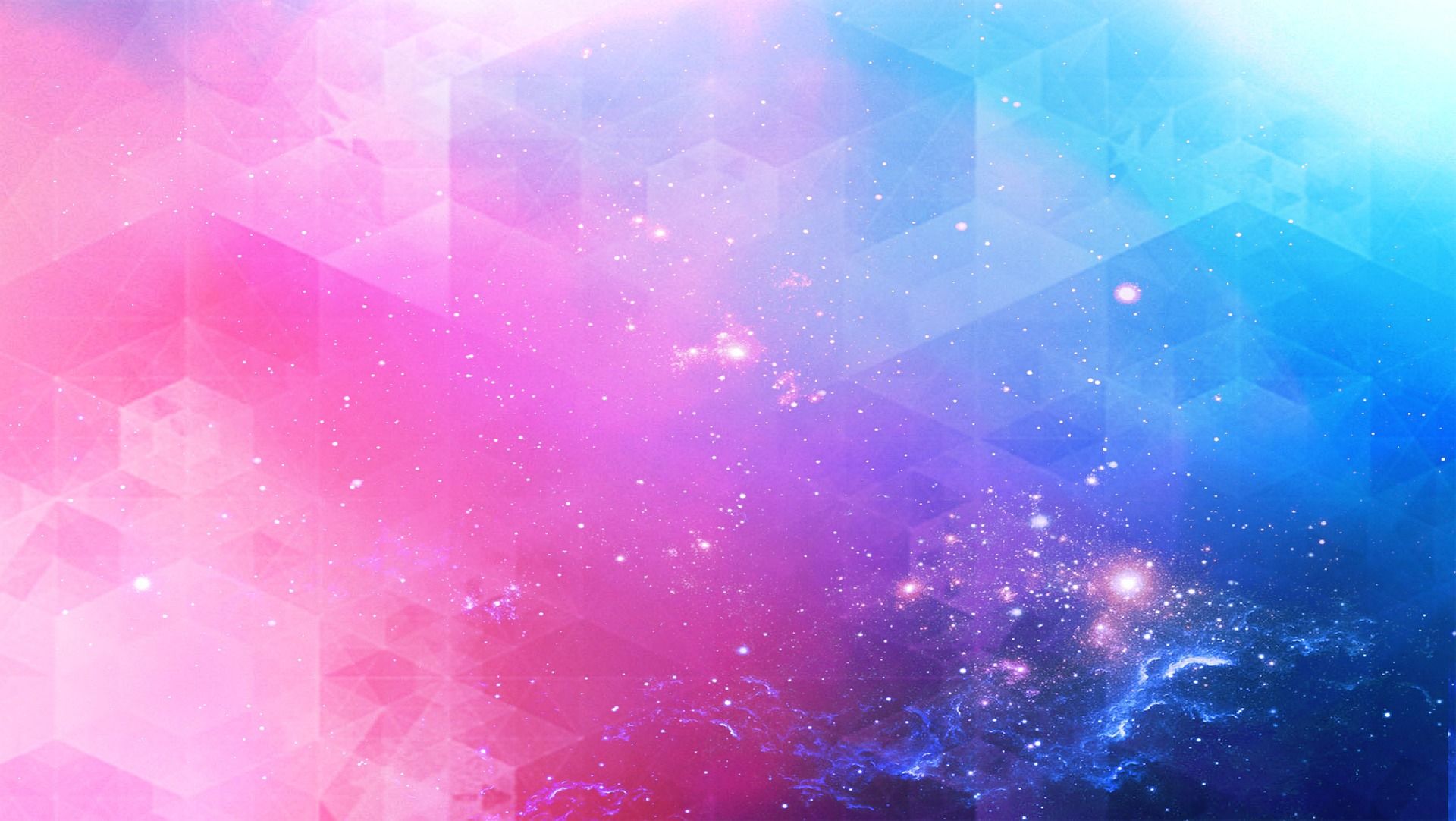
In a recent Wall Street Journal article, the author highlighted how venture capital firms have long been funding AI companies, although they're now starting to use the technology to guide their own investment decisions.
Although many VCs see the opportunity of using data science and machine learning in their investment process, the application of AI in venture capital is still in the early stages. In particular, Gartner says that less than 5% of venture capital investment decisions utilize data science and machine learning, although that number is expected to reach 75% by 2025.
As Patrick Stakenas, senior research director at Gartner says:
Successful investors are purported to have a good “gut feel” — the ability to make sound financial decisions from mostly qualitative information alongside the quantitative data provided by the technology company.
In this article, we'll look at who is currently using AI and machine learning in the venture capital industry, how the technology can be applied to guide early-stage investing, interesting datasets that machine learning engineers can use, and several papers on the topic.
Stay up to date with AI
We're an independent group of machine learning engineers, quantitative analysts, and quantum computing enthusiasts. Subscribe to our newsletter and never miss our articles, latest news, etc.
Companies Using AI & Machine Learning in VC
To start, let's first look at the companies pioneering the application of AI and machine learning in venture capital.
Correlation Ventures
The WSJ article mentions several VCs already using AI to distribute capital, of which Correlation Ventures is one of them. The firm has $365M under management and, with the help of its models, is able to make investment decisions in under two weeks.
According to David Coats, co-founder and managing director, the firm use machine learning to determine whether or not to invest in a business. The company also only invests in funding rounds where a lead investor is present.
The machine learning tool they use, which was built in-house, analyses data collected by humans in the form of pitch decks and other startup materials.
Coats clarified that the data is fed into an algorithm that has been trained on data from over 100,000 venture capital rounds. The algorithm determines how variables like team experience and board composition affect potential investor returns.
The AI tool, which assigns a score to each startup, is intended to make the investment process go faster. As Coats said, "We commit to making investment decisions in under two weeks, but we have done so in less than 24 hours."
EQT Ventures
According to Stockholm-based EQT Ventures partner Henrik Landgren, the company uses an internally developed AI framework called "Motherbrain" to guide its employee workflows.
The platform is built on a proprietary database that includes data such as startup financials, site traffic, and team member job history. Landgren explained that it rates investment opportunities on a scale of 1 to 340. The highest-ranking opportunities will then be investigated first by investment professionals.
According to Landgren, the organization made four investments from its first fund that would not have existed if it hadn't been for Motherbrain. Peakon, one of their investments, was sold to Workday Inc. earlier this year, and Landgren said that the profit from that transaction essentially paid for the other three investments. It will be "complete upside" if the other three investments also yield positive returns, he said.
Sapphire Ventures
Sapphire Ventures, a Palo Alto, California-based company with over $5.7 billion in assets under management, is considering using artificial intelligence to make forecasts for its current portfolio companies.
Sapphire's president and associate, Jai Das, believes AI will not be able to replace human judgment, instead, he told the WSJ:
I think the gut is never going to go away, but I think it’ll be much more driven by data and analysis than before. And you’ll have data to show that people who say I’m voting with my gut, either they’re right or not.
Hone
Hone is another VC first that partnered with AngelList in order to create a machine learning algorithm based on 30,000 deals in the past 10 years. As Coresignals highlights, the data was sourced from AngelList, Pitchbook, Crunchbase, and MatterMark.
From this dataset, they were able to analyze more than 400 characteristics such as funding raised, the founder's background, conversion rates, and more. These characteristics were then used to rank the top 20 companies with the highest potential for success.
Applications of Machine Learning in Venture Capital
As the above examples of companies already using AI in venture capital highlight, there are a few different ways that machine learning can improve the startup funding research and investment process.
Below are 5 applications and benefits of machine learning in venture capital highlighted by the alternative data company CoreSignal.
1. Discovering companies seeking funding
One of the most obvious applications of machine learning is for screening a large number of startups. VCs can use machine learning to filter companies based on their preferences and then output a list of the startups that fit those criteria and have the highest potential for future success.
2. Identifying early growth signs
Machine learning can also be used to analyze vast quantities of public data such as site traffic, social media mentions, and so on to identify early signs that a company is growing rapidly. Public data could also be used to analyze founders and other professionals and identify if they've just started a new company, for example.
3. Investment timing
Similar to identifying growth signs, machine learning can also be used to determine the right timing of an investment based on the fund's criteria and the company's early growth indicators. For example, analyzing trends in company recruiting and hiring strategy could indicate the speed of their growth.
4. Tracking growth of portfolio companies
In addition to tracking the growth of young startups, VCs can also use machine learning to analyze the growth of their existing portfolio companies, their companies, and the industry in general. A few examples include online review monitoring, sentiment analysis from social media, and analyzing trends in their online ad spend.
5. Employee satisfaction tracking
Finally, machine learning can be used to track and analyze employee satisfaction in portfolio companies and competitors alike. With the recent difficulties many companies are having in hiring talent, employee satisfaction is a key indicator of a company's future growth potential and management effectiveness.
Venture Capital Datasets
Now that we've looked at a few applications of AI and machine learning in venture capital, let's review a few relevant datasets that could be used for training machine learning algorithms.
In the WSJ article, they mention that Correlation Ventures trained their AI on data from 100,000 financing rounds, so in this section, we'll look at various VC and PE datasets that could be used for training a model.
Crunchbase
With over 625,000 companies, Crunchbase Data is one of the most comprehensive VC databases. The platform offers developers either REST API access or a daily CSV export that can be used either in an application or for analysis and model building.
Pitchbook
Pitchbook is another one of the most widely used databases for VC and private equity data. With their API you've got access to more than enough private market data to build an ML model.
CB Insights
CB Insights is a tech market intelligence platform that analyzes millions of data points on venture capital, startups, patents, and investor activity. In addition to their massive database, CB insights also uses natural language processing (NLP) to analyze text data, data visualization, and predictive analytics to predict emerging trends.
Coresignal
Finally, since there is usually less financial data available on early-stage companies, VCs are often making investments based on the experience of the team. In order to build models based on the people behind the company, Coresignal is an interesting alternative data provider that provides data on millions of professionals globally.
VC & ML Papers
Now that we've looked at a few datasets that could be used, let's review a few interesting papers on the topic of machine learning in venture capital.
CapitalVX: A machine learning model for startup selection and exit prediction
This paper uses a dataset from Crunchbase to develop an ML model they call CapitalVX, which predicts the outcomes of startups, i.e. whether they will successfully exit through an IPO or acquisition, fail, or remain private. Their model was able to achieve an out-of-sample accuracy of startup outcomes between 80-89%. As the authors write:
This research suggests that VC/PE firms may be able to benefit from using machine learning to screen potential investments using publicly available information, diverting this time instead into mentoring and monitoring the investments they make.
Assessment of Machine Learning Performance for Decision Support in Venture Capital Investments
Instead of simply trying to predict two classes of exists—being acquired or IPOing—this paper ties to predict more possible startup outcomes including future funding rounds or the closure of the company. The paper uses a dataset of over 120,000 companies and analyzes the performance of several machine learning algorithms to predict the performance over a 3-year time period.
This paper seeks to predict valuation step-up multiples in subsequent funding rounds of VC-backed US companies using data from Pitchbook. The authors use a regression-based model and a fully connected 10-layer neural network to predict future valuation step-ups. Their results showed that deep learning techniques did outperform statistical inference models such as linear regression.
Summary: AI and Machine Learning in Venture Capital
In summary, it's clear that the venture capital investment process will shift from a largely manual, gut-driven approach to incorporate data science and machine learning in some capacity. Given the access to massive datasets of early-stage companies mentioned above, it makes sense that machine learning methods can improve VC investing by filtering companies, uncovering new opportunities, and tracking existing portfolio companies. If Gartner's prediction is correct that 75% of all venture capital investment decisions will use data science and machine learning by 2025, this clearly presents a massive shift and opportunity in the industry that will be interesting to watch unfold.